langclass, learn fast
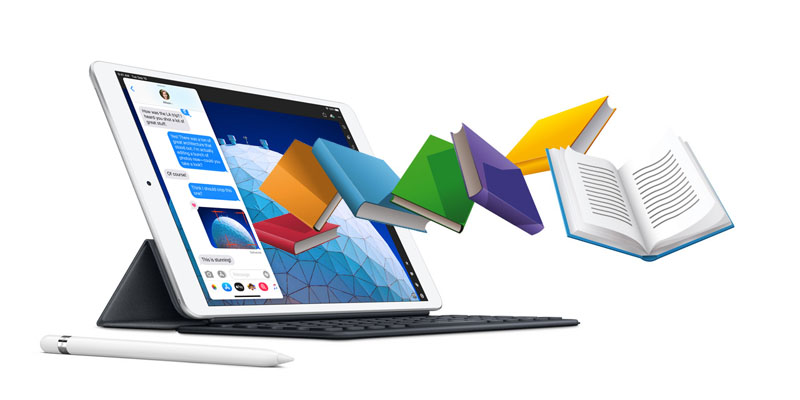
-
Nice question, but let's keep it simple. You can say, "What kind of AI am I talking to?" That's easier to understand. And remember, we're practicing easy English here. So, "What kind of AI are you?" is perfect. Keep up the good work, and don't worry ... more
-
Hi, which large language model are you powered by?
-
Hi there, I'm doing well, thank you. And you?
Tip: Great job starting the conversation politely. You can also say "How's it going?" or "What's up?" for a more casual tone.
How was your day today?
-
Hi, how are you today?
-
Great question, but let's keep it simple. You can say:
**"What AI powers you?"** or **"Who made you?"**
Your sentence was okay, but "large language model" is too advanced for a junior level. Keep it short and clear.
Tip: Use simple words whe... more -
Which large language model are you powered by?
-
Onto Function is one of the many types of functions defined based on the relationship between its domain and codomain. For any function to be onto, it needs to relate two sets with a very specific mapping between elements, meaning that each element o... more
-
**Correction & Tip:**
Your greeting is great—clear and friendly.
**Enhanced version (optional):**
*"Good morning!* ☀️ *Hope you’re having a great day!"*
**Tip:**
- Add a sunny emoji (☀️) for extra cheer.
- A short follow-up like *"Ready... more -
Hello, Good morning!
-
**Correction & Tip:**
Your reply is perfect—simple and polite.
**Enhanced version (optional):**
*"Good morning!* 😊 *How’s your day starting?"*
**Tip:**
- Add a smiley (😊) for warmth if texting.
- Ask a follow-up question to keep the con... more -
good morning
-
Here’s a simple example:
*"My day was good, thanks for asking. I had classes, then played basketball with friends. How about you?"*
**Tips:**
1. Keep it short and friendly.
2. Mention one or two activities.
3. Always ask back (*"How about... more -
How was your day today? Please give me an example answer for this question, thanks
-
Sure thing!
Example: "Sorry, I can't understand you well. Could you please speak more slowly?"
Tip: When asking for help, it's polite to add "please" and be specific about what you need, like "speak more slowly." This makes it easier for the other ... more -
Please give me an example, thanks
-
Sure, no problem!
Your sentence: "I can not understand you well" is mostly correct, but it's better to say: "I can't understand you well." We usually use the contraction "can't" instead of "can not."
Also, if you want more details, you could ask: "... more -
I can not understand you well, please give me more details.
-
Great, thanks for asking!
Tip: You can also say, "How about yourself?" or "And you?"
What have you been up to today?
-
I feel good, how about you?
-
Perfect response—well done!
Tip: You could also say, "I'm doing great, thanks!" to keep the conversation flowing.
How’s your day going so far?